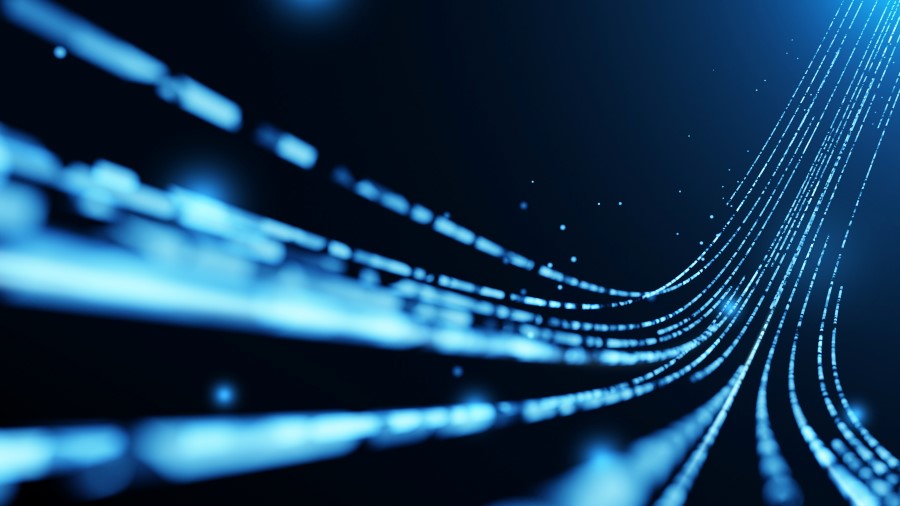
Data Integration – A Complete Guide
In today’s digital age, businesses are generating massive amounts of data from various sources, such as customer transactions, social media, website analytics, and more. This data is a goldmine of insights that can help organizations make informed decisions, improve customer experiences, and drive growth.
Table of Contents
However, the challenge lies in how to integrate this data from disparate sources and formats into a unified, usable format that can be analyzed and acted upon. This is where data integration comes in.
Highlights:
What is Data Integration?
Data integration is the process of combining data from different sources and formats to create a unified view. This includes bringing together data from various databases, data warehouses, cloud applications, and other data sources and transforming it into a consistent, usable format. Integrating all this information into a single, cohesive format enables businesses to gain a better understanding of their operations, customer behavior, and market trends.
A properly designed data integration strategy can save IT costs, free up resources, and foster creativity without requiring major changes to the applications or data structures in use. Businesses with expertise in data integration enjoy a significant competitive advantage, including:
What are the Different Types of Data Integration?
There are four types of data integration, each with its unique advantages. Businesses must choose the type that best suits their needs based on the volume and complexity of data, budget, and infrastructure.
Manual Data Integration
Manual data integration involves manually combining data from different sources, often using tools like spreadsheets. This type of integration requires a lot of human effort, so it can be time-consuming, labor-intensive, prone to errors, and expensive. However, it can be effective for small-scale data integration projects, where the data sources are relatively simple or not easily accessible through automated methods, and the volume of data is low.
Application-based Data Integration
Application-based data integration is the process of using APIs (Application Programming Interfaces) or web services to extract, transform, and load data from various sources. This method is often used for integrating data between different applications or systems, such as CRM (Customer Relationship Management) and ERP (Enterprise Resource Planning) systems. It is more efficient than manual data integration since it can automate many manual processes, reducing the time and resources required. This approach can benefit organizations using multiple software applications or systems that need to be integrated.
Middleware-based Data Integration
Middleware-based data integration involves the use of a middleware layer to manage the integration of data between different systems. The layer acts as a bridge between different systems, allowing seamless data transfers. This type of integration can be useful when dealing with large amounts of data or when integrating data from disparate systems. It can help to reduce the complexity of the integration process and make it easier to manage.
Cloud-based Data Integration
Cloud-based data integration allows businesses to access and integrate data from various cloud-based applications and services. This is becoming increasingly popular due to its scalability, cost-effectiveness, and flexibility. It helps businesses to easily and quickly scale their data integration projects per changing needs and take advantage of the latest tools and technologies without having to invest in expensive infrastructure.
What is Data Warehousing?
Data warehousing is the process of collecting, storing, and managing data from a variety of sources in a centralized repository to be optimized for efficient querying and analytics. This data is typically historical, integrated, and non-volatile and serves as a basis for business intelligence (BI) and decision-making. It encompasses a range of critical sources like transactional systems, operational databases, and external data sources.
Most tools for data integration, such as ETL and data virtualization, may also involve data consolidation and transformation, but they are primarily focused on integrating real-time or near-real-time data from various sources into operational applications and workflows. These types of integration are often used to support transactional and operational systems, while data warehousing is designed for analytical processing and decision-making.
Note: Data warehousing is a type of data storage and management system rather than a type of data integration. However, data warehousing can be used in conjunction with data integration to provide a more comprehensive solution for managing and utilizing data.
How is Data Integration Performed?
A well-defined integration procedure ensures the quality and accuracy of the integrated data. This consists of:
Step 1: Data Profiling
It involves analyzing the data sources to identify potential data quality issues. Data profiling helps in identifying inconsistencies and errors in data and enables the integration team to take corrective measures.
Step 2: Data Cleansing
Once data profiling is complete, data would need to be cleaned. This step involves identifying and correcting data quality issues, such as missing values, incorrect formatting, and inconsistencies.
Step 3: Data Mapping
In this step, the data from the source system is mapped to the target system while ensuring there is no data loss or corruption.
Step 4: Data Transformation
Data transformation is the conversion of data from one format to another, making it compatible with the target system. This step is crucial in ensuring the data can be used effectively in the target system.
Step 5: Data Loading
In this step, the transformed data is loaded into the target system. It is important to ensure that the data is loaded correctly and there are no errors or inconsistencies.
Step 6: Data Validation
This is the final step in the data integration process. It helps ensure the data is complete, consistent, accurate, and reliable.
Tools and Technologies
Data integration tools are essential to automate and streamline the data integration process. Some of the most widely used tools and technologies are:
Extract, Transform, Load (ETL)
ETL is a data integration process that extracts data from multiple sources, transforms it into a common format, and loads it into a target database. ETL tools provide a graphical interface for designing and managing data flows, handling large volumes of data from different sources, and automating the process. It ensures data quality by cleaning and standardizing data during the load process, making it an efficient way to keep it up to date and in sync across different systems.
Enterprise Service Bus (ESB)
ESB is the middleware that centralizes communication, management, and control of data flows between different applications and systems, even with varying protocols and formats. It enables message-based communication and has advanced features such as routing, data transformation, and protocol conversion. ESB is useful for complex and heterogeneous integrations, improving the messaging infrastructure’s reliability, security, and scalability.
Data Virtualization
Data virtualization integrates data from multiple sources without physically moving or transforming it. This technology presents data from different sources as if it were in a single location, providing real-time access without requiring a data warehouse or ETL process. This agile and flexible approach to data integration is ideal for real-time needs and well-suited for organizations that require timely and dynamic data.
Master Data Management (MDM)
MDM is an approach to data integration that creates a single source of truth for shared data across the enterprise. It includes customer, product, and financial data that is accurate, consistent, and up-to-date. MDM processes and technologies involve data quality, data models, and specialized tools for data profiling, cleansing, and enrichment. This approach requires coordination across different business units and departments in the organization to maintain the data.
What Benefits Does Data Integration Provide?
The benefits of data integration are numerous and far-reaching for businesses of all sizes and industries. It is the key component of any modern data-driven organization, enabling better decision-making, increased efficiency, and improved business outcomes. It can positively impact a company’s bottom line through:
Improved Data uality
By integrating data from various sources and standardizing it, businesses can eliminate duplicate or inconsistent data.
Enhanced Decision-making
Businesses can develop a single source of truth for all partners to engage with and contribute to by integrating their data. This assures company leaders that the information they are using to make choices is always up-to-date and comprehensive
Increased Productivity
Data integration can streamline data processes, reducing the need for manual data entry and other time-consuming tasks. This can free up resources to focus on more strategic activities.
Cost Savings
By eliminating data silos and streamlining data processes, businesses can save time and money on data management. In addition, integrated data can help businesses identify cost-saving opportunities and optimize their operations.
Data Integration Use Cases
Data integration has a wide range of use cases that can bring significant business value to organizations. Some of the most common use cases are:
Mergers and Acquisitions
When two or more companies merge, they usually have different IT systems and data sources that need to be integrated to avoid any disruption to business operations. Data integration can ensure that the data is consolidated and made available in a uniform way.
Customer 360
Data integration can be used to combine customer data from various sources like sales, marketing, and customer service systems. It is then used to create a 360-degree view of the customer, which can be used to provide personalized experiences and improve customer satisfaction.
Supply Chain Management
Data integration can be used to integrate data from different suppliers, partners, and logistics providers to get a unified view of the supply chain, better manage operational flow, and reduce costs.
Data Migration
When companies switch to new IT systems or cloud platforms, data integration can help migrate data from legacy systems to the new platform seamlessly and efficiently.
Big Data Integration
With the growing volume and complexity of big data, it has become critical for businesses to extract better insights and enable faster decision-making. It involves the integration of data from various sources, such as social media, IoT devices, and enterprise systems, to create a unified and complete view of data for analytics and reporting.
Data Quality Management
Data quality management includes data profiling to identify data issues, data cleansing to remove errors and inconsistencies, and data validation to ensure data accuracy and completeness. With this practice, businesses can ensure that they are working with reliable and trustworthy data.
Challenges of Data Integration
Data integration is a critical component of modern business operations, enabling organizations to make better decisions, improve productivity, and reduce costs. However, it is not without its challenges, and organizations must be prepared to tackle them to succeed. Some of the key challenges include:
Data Incompatibility
One of the biggest challenges with data integration is dealing with incompatible data formats. Different systems may use different data structures and data types, making it difficult to integrate data from these systems. Organizations must invest in tools and technologies that can help them convert data from one format to another while ensuring quality and consistency.
Data Security and Privacy Risks
Data integration involves the exchange of data between different systems, which can pose security and privacy risks. Organizations must implement robust security measures, including access controls, encryption, and secure transmission protocols, to ensure that data is not compromised during integration.
Lack of Data Governance
Data governance is key to successful data integration as it involves creating and enforcing policies, procedures, and standards to manage data throughout its lifecycle. Without a clear framework, inconsistencies in data quality, security vulnerabilities, and lack of data standardization can impede integration efforts. Achieving collaboration and buy-in from stakeholders is also challenging without clear leadership and communication. Therefore, a robust data governance framework is critical for long-term data value.
Integration Complexity
Data integration can be a complex process, particularly when dealing with large volumes of data or multiple data sources. Organizations must invest in tools and technologies that can simplify the integration process and automate as much of it as possible. They must also be prepared to deal with unexpected challenges that may arise during the integration process.
Best Practices
Organizations can implement some time-tested practices to maximize the value of their data and improve their ability to make informed decisions based on accurate and reliable data. They include:
Developing a Clear Strategy
Developing a clear strategy is critical for the success of any data integration project. A clear strategy will help identify the goals and objectives of the project, define its scope, and determine the best approach to achieve the desired outcomes. The strategy should also consider the existing data landscape and ensure the integration approach aligns with the overall business strategy.
Implementing Data Governance
Data governance is essential to ensure that the integrated data is accurate, consistent and meets the desired quality standards. Implementing a data governance framework involves defining policies, procedures, and standards for data management and establishing accountability for data quality. It is also essential to have a team in place to oversee the data governance framework and ensure compliance with the established standards.
Using Standardized Data Formats
Using standardized formats is critical for ensuring that data is consistently structured and can be easily shared across different systems and applications. They include data models, schema, and other formats that help ensure data consistency and integrity. Organizations can save money and time by employing standardized formats while guaranteeing the accuracy and dependability of the integrated data.
Implementing Data Security Measures
Implementing data security measures involves identifying potential risks and vulnerabilities in the data integration process and developing strategies to mitigate them. This may involve data encryption, access controls, and other security measures to protect sensitive data. It is also important to ensure that data is backed up regularly and that disaster recovery plans are in place to minimize the risk of data loss or corruption.
Conclusion
The future of data integration looks promising with the emergence of several exciting trends. The increased adoption of cloud-based data integration is expected to continue as more businesses realize the benefits of cloud-based solutions, including scalability, flexibility, and cost-effectiveness. The emergence of AI-enabled integration modules is expected to revolutionize the field, enabling faster and more accurate results, with the potential to automate many of the manual processes involved. The integration of structured and unstructured data is becoming increasingly important as businesses seek to gain insights from a broader range of data sources. Overall, these trends suggest a future where data integration becomes more seamless and efficient, enabling businesses to make better use of their data and gain a competitive edge.
About Intellicus
Intellicus is a business intelligence and analytics platform that offers several data integration services and products that aim to help organizations easily connect and integrate their data from various sources. It offers a comprehensive suite of products and services, including a powerful ETL tool and a cloud-based data integration platform, Intellicus Cloud. In addition, Intellicus offers a range of integration services, including data profiling, cleansing, mapping, and validation, to ensure that data is accurate, complete, and consistent. With its end-to-end solutions and expertise in data integration, Intellicus is well-positioned to help organizations efficiently and effectively manage their data and gain valuable insights to make informed decisions.
Take your data integration to the next level with Intellicus. Reach out now to learn how it can help your organization achieve efficient and effective data management.