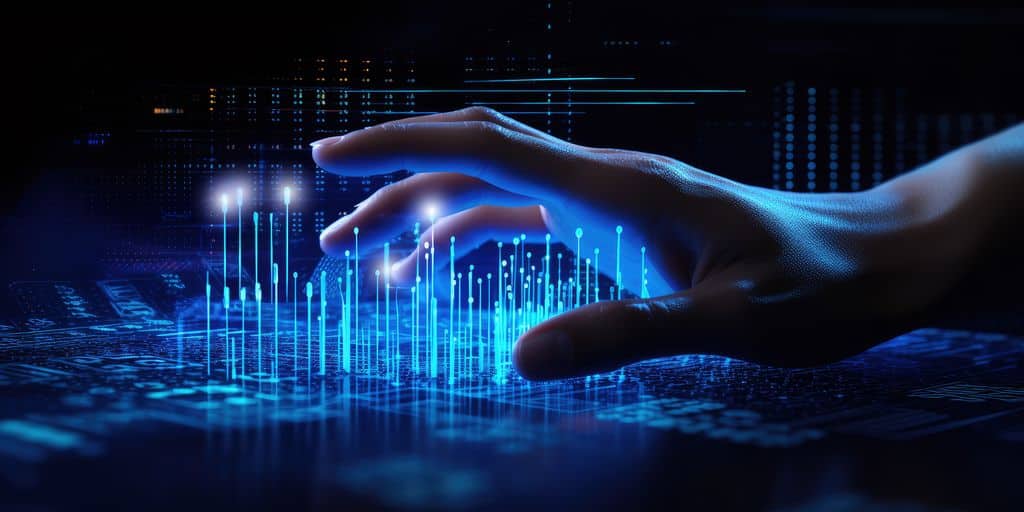
Data Visualization: A Gateway to Clarity and Insight
Imagine standing in front of a 3-story building at night. The lights in apartment windows make it look much like a small spreadsheet, don’t they? With each window representing a data cell, this small spreadsheet contains a limited amount of data which is easy to comprehend at a glance.
Table of Contents
Now, think about standing at the base of a 100-floor skyscraper. Consider how difficult it would be to see all its details from the base to the top, in one view. Observing the full skyscraper without moving far back or going high enough seems utterly impossible. This is what it is like to make sense of a massive and complex dataset.
Having data management tools is like having a zoom lens for observing that skyscraper. They allow the viewer to zoom in and focus on specific floors or zoom out and see a panoramic view of the entire building. Data visualization takes this process even further by using computing techniques to model complex events and visualize phenomena that cannot be observed directly.
What is Data Visualization?
In today’s data-driven business culture, organizations look to collect data on every possible interaction. Such large-scale collection makes it essential for data engineers to devise tools that present the data clearly and effectively. Data visualization transforms data into graphical representations like charts, graphs, maps, dashboards and reports. The concept relies on the human species’ cognitive abilities to understand visual signals and aims to simplify pattern recognition and improve memory retention.
In the absence of sufficient clues, data stored in huge tables can confuse the human mind. Therefore, such data needs to be processed further to identify shared attributes between the different data points. Data visualization tools can then club the data based on their common attributes and use visual elements (like maps or graphs) to represent it in an abstract yet accurate illustration. The technique aims to reveal hidden patterns, trends and insights to improve decision-making and convey data-driven narratives comprehensibly.
Key Elements of Data Visualization
Data visualization is a perfect synergy between the science of data and statistics combined with the art of design and presentation. Preparing visual diagrams from complex datasets requires a keen sense of aesthetics and a deep understanding of cognitive perception.
Data points: Visualized data points serve as individual information units, making the information more meaningful. They support or disprove hypotheses, spot trends or patterns, make future predictions and observe changing trends. Each data point corresponds to a specific value or set of values whose relative arrangement can convey patterns, trends and relationships in the data.
Visual elements: Data points are turned into visualizations using a combination of visual elements such as lines, shapes, colors, textures and forms. These elements are essential for creating effective and engaging visualizations using the design principles of balance, gradation, repetition, contrast, harmony, dominance and unity.
Axes and scales are important because visualizations need to fit on a screen. Most visualizations have two axes: x and y. The horizontal x-axis usually represents the independent variable, while the vertical y-axis represents the dependent variable. Scales are added at regular intervals on both axes. The data is then plotted between the two axes. The charting coordinates are decided as per their frequency or magnitude.
Process of Data Visualization
Data visualization is an intricate process to ensure that along with being informative, the data is also engaging and insightful.
Define objectives: Clearly defining the goals and objectives guides the entire visualization process. It ensures that the visualizations effectively serve their intended purpose, aiding in identifying trends, comparing values, uncovering insights or communicating specific information to the target audience.
Choosing the right visualization type: Consider a range of factors such as the nature of the data (categorical or numerical), the interrelationships between variables and the intended narrative. By doing so, determine the most suitable visualization type that best represents the data’s essence and communicates the story effectively.
Designing the visualization: After selecting the appropriate visualization type, create the visual representation using visualization tools or software. Focus on design principles, including color schemes, layout, font choice, labeling and consistency. This meticulous design process ensures that the visualizations are clear, visually appealing and easily interpretable by the audience.
Presentation and sharing: Prepare the visualizations in various presentable formats for sharing with the intended audience. Develop comprehensive reports, interactive dashboards, engaging presentations or informative infographics that effectively deliver the insights derived from the data. Tailor the presentation to match the audience’s level of understanding for enhanced accessibility and comprehension.
Feedback and iteration: Gather valuable feedback on the visualizations from stakeholders or end-users, using it as a basis for iterating on the design or presentation of the visualizations. Address concerns, refine elements requiring improvement and ensure that the visualizations align with the audience’s expectations and objectives.
Continuous monitoring and updates: Regularly monitor changes in the data and update visualizations as necessary to reflect the latest information or insights. This helps them remain relevant, accurate and informative over time.
Various Types of Data Visualization
Sophisticated data management and visual design tools actively work together to transform large datasets into visual narratives. The visualization can be something as simple as a chart or a graph to anything as advanced as geospatial maps or interactive dashboards. These tools support pattern recognition, insight extraction and decision-making for professionals across various industries. Every visualization serves a unique purpose to meet specific analytical needs and enable data consumers to understand the presented data for any context and application.
Basic charts
Apart from being the earliest tools for data visualization, basic charts are widely popular for their simplicity and effectiveness in conveying data. Below are the most commonly used chart types:
Bar charts: These visualizations ideally compare quantities across various categories. Varying representations of vertical bar charts, horizontal bar charts, stacked bar charts, etc., help visually analyze business sales data, revenue comparisons, periodic performance trends and a lot more.
Pie charts: Standard pie charts and donut charts can show proportions and percentages in situations like market share analysis, budget allocations, etc.
Line graphs: Various types of line graphs effectively display data trends over time. The most popular use cases are stock market trends, website analytics, defect rates over time, etc.
Advanced charts
Advanced charts meet more complex data visualization needs, offering a deeper insight into data.
Histograms: These charts show the distribution of a dataset while understanding the frequency of customer age groups, distribution of products purchased, etc.
Scatter plots: Excellent for showing the relationship between two variables; they represent correlations between advertising spending and sales, year-over-year growth numbers, etc.
Area charts: These charts are similar to line graphs but emphasize volume or magnitude. The data with a higher value gets a larger area on these charts to track demographic changes over time, website traffic sources, etc.
Infographics
Infographics combine visuals with data to present information engagingly and informatively.
Statistical infographics: This combination of charts and graphs focuses on data and statistics to present annual reports, market research summaries, etc.
Informational infographics: They provide a quick overview of a topic with a mix of visuals and text.
Timeline infographics: These infographics serve the purpose of displaying a list of events in chronological order. Timelines are used extensively on company websites and project brochures where they present chronological concepts like company history, project milestones, process workflows, etc.
Geospatial Maps
Geospatial maps play a vital role in visualizing data with a geographical component, placing data in a spatial context. However, this category of visualizations finds applications in various other disciplines other than geography and meteorology.
Heat maps: Heat maps display the density or intensity of variables in different geographic areas, pattern recognition in financial markets and other information-dense environments.
Choropleth maps: Choropleth maps use colors and shades to differentiate data values in predefined areas while observing sales by region, product distribution by area, etc.
Bubble maps: Bubble maps represent data values in specific locations. The sizes of circles change depending on the frequency, volume or concentration of the data. For example, visualizing market size in different regions.
Interactive Visualizations
In use cases where organizations want to allow users the freedom to engage with the data, they use these visualizations to offer a dynamic way for complex data analysis.
Dashboards: Business intelligence extensively uses dashboards, combining multiple visualizations into a single interface. The same data or mutually related datasets can be observed from various perspectives using different visual representations.
Data exploration tools: These tools enable users to interact with the data, often with options to filter and drill down into specifics, especially in financial market analysis, market research, etc.
Interactive reports: Interactive reports introduce an interactive element into traditional reports, such as annual business reports, interactive portfolios, etc.
Advantages and Disadvantages of Data Visualization
Data visualization is a powerful tool in the modern data-centric world, offering a range of benefits while also posing certain challenges. Understanding these can help in maximizing its effectiveness while mitigating potential drawbacks.
Advantages
Improved understanding: Rapid advancements in the field allow data visualizations in different formats to make them discernible for various audiences. Visuals make it easier for people to observe patterns, trends and outliers, which might go unnoticed in spreadsheets.
Enhanced decision-making: As specified above, if users can understand data easily, they can also make decisions based on that data. Additionally, visual cues in the illustrations can make it easy to identify behaviors hidden in the data and predict the direction it will take in the future.
Accessible insights: Non-technical users do not have a map to the vast data repository at their disposal. Hence, plotting the data using various designs can help draw their attention to the right data points.
Pattern recognition: The process consolidates the data in homogenous categories. This representation can highlight trends and relationships in data sets that might be difficult to decode with traditional analysis methods.
Customization: With high-performing servers and advanced processing capabilities, modern visualization tools come equipped with customization capabilities. Along with aesthetic elements like colors and shapes, users can also change the format of visualizations and produce variations of the same data for different focus groups.
Hypothesis testing and discovery: In science and business, there are educated assumptions that need to be validated with data. Once the hypothesis is confirmed, data visualization can lead to more robust research and discovery.
Disadvantages
Risk of misinterpretation: If the intent was X and the data ends up communicating Y insights to the audience, it can really hurt the interests of the data owner. Poorly designed visualizations can lead to the audience arriving at unintended conclusions, especially if they lack the expertise in interpreting the data.
Oversimplification: Simplified representation of complex datasets can sometimes border on generalization. Such oversimplification of data can lead to the omission of important nuances and details. Decisions can be compromised and impact on the future of the project.
Technical requirements: While spreadsheet software now comprises basic charts and graphs, creating advanced data visualizations still requires technical knowledge. The business teams need to enlist the help of design and IT experts to execute detailed data analysis.
Time-consuming: Logic definition can be a serious hurdle in the process of visualization. Data analysts must devise the right algorithm to represent the data accurately.
While data visualization is an incredibly powerful tool for understanding and communicating data, it has its own challenges. Someone still learning the ropes needs to realize the importance of approaching the process with a critical eye, ensuring that the visuals produced are as accurate and effective as possible.
The Importance of Data Visualization
With decreasing decision deadlines and increasing number of variables, data visualizations are becoming necessary for business understanding. Leaders are required to make split-second decisions based on complex metrics and densely populated spreadsheets, which is why data visualizations matter so much. When making broader decisions about wider groups, managers can access consolidated reports, graphs or charts to look at the bigger picture. Similarly, they can drill down to the most minuscule details for increased attention to detail.
Business intelligence platforms like Intellicus prepare customized dashboards for real-time access to business KPIs, enabling constant monitoring of the organization’s health. While creating a single source of truth for the entire organization, the platform delivers relevant insights to specific functions. With robust data governance frameworks, department-specific dashboards can be generated while also maintaining a holistic view for the top management.
Further, data visualizations promote data storytelling, which is especially important in strategy meetings. Decision-makers can extract real-world business insights and discover the latest trends. They can identify operational anomalies and look for solutions to optimize productivity. In essence, storytelling helps drive action-based activities, rather than a superficial exchange of superficial ideas.
Data visualizations can promote a data-driven culture within the organization by achieving all this. When every business interaction is based on factual information, the workforce learns new ways to value and utilize data. With data democratization, employees feel empowered to actively participate in discussions backed by tangible facts and figures. When the entire organization speaks the language of data, results can be quantified and evaluated effectively.
Categories Of Data Visualization
If data has categories, data visualization should too. Businesses can choose category-specific visualizations to represent the type of data meant to be visualized. Here are the categories of data visualizations based on data types:
Quantitative data visualizations: Quantitative or numerical data visualizations represent amounts or quantities. These visualizations are generally used for large datasets containing raw numbers.
Continuous data: When the data is populated with measurements on a continuous scale, these visualizations are used to show trends over time, distribution of continuous data, cumulative totals over time or relationships between continuous variables. Examples of quantitative data visualizations include line graphs, histograms, scatter plots, area charts, etc.
Discrete data: Countable items that can only take certain values fall under discrete data. The visualizations for this category can compare discrete quantities across categories, represent percentages or proportions of discrete data or visualize three-dimensional data.
Qualitative data visualizations: Also known as categorical data visualizations, the data for this category generally represents groups. These visualizations represent categorical variables showing characteristics such as demographics, process mapping, workflow analysis, etc.
Binary data: This is a special type of categorical data that has only two categories of outcomes like yes or no, true or false, etc. Customer satisfaction surveys with binary scales like satisfied vs. unsatisfied are a popular business use case.
Nominal data: These visualizations display the number of data points falling in different categories. For example, using bar charts to display the number of customers in different demographics.
Ordinal data: This category of visualizations is used to represent project timelines or business processes in order of their completion. Company timelines on websites are a prime example for this type.
Various visualization techniques are tailored to each data category. Businesses can transform raw data into actionable insights to drive growth and innovation.
Data Visualization and Big Data
Big data visualization is becoming increasingly important for advanced data analysis and decision-making. IT and management often design visualization tools to help stakeholders understand big data by examining patterns and trends.
Predictive and machine learning algorithms make visualizations more comprehensible than numerical results. Interactive reports allow users to interact with the data by drilling down to the lowest granularities or transforming its shape. With this ability, business users can make in-depth discoveries by posing unexpected questions on the spot.
Real-time visualization becomes crucial in dynamic environments such as financial trading and network monitoring because it offers up-to-date and real-time insights. These advanced visualizations have allowed organizations to derive useful information from their big data for faster decision-making, thus creating a competitive edge in the market.
Another important characteristic of big data visualization involves integrating multiple data sources. Such integration empowers organizations to discover commonalities between data from diverse sources and gather insights from the data silos that might influence comprehensive analysis.
Furthermore, the rise of big data has prompted the development of specialized visualization techniques for specific types of data. It is a prominent area of active R&D in the field of data management.
Artificial Intelligence (AI) and Machine Learning (ML) Integrations in Data Visualization
In the age of AI and ML, data visualization brings a revolutionary transformation in the understanding of complex data sets. AI and ML algorithms can automate data analysis and visualization by mining through large data sets to pick out patterns, trends and oddities. The algorithm can automatically choose the most appropriate visualization based on data distribution and category correlations.
The application of such new technologies can further customize visualizations to suit individual users’ interactions and choices, emphasizing the most important inferences. They also perform very well when handling real-time data, enabling live visualization of data streams. It can identify production line faults in supply chain management, network disruptions in telecommunication, customer behavior anomalies in e-commerce, etc. for rapid response and preventive measures.
Going beyond its static capabilities, AI-powered charts and dashboards also provide dynamic and interactive visualizations that adapt to real-time updates and varying data streams. These tools enhance scalability by automating repetitive tasks, allowing data scientists to work on more complex problems and efficiently handle larger datasets without significant manual intervention. Additionally, automated evaluation metrics built into these systems ensure that the visualizations meet specific performance requirements.
Moreover, the emphasis on interpretability, bias reduction and error handling contributes to more trustworthy and fair AI systems. By significantly reducing manual intervention, they enhance efficiency and enable scalability.
Measuring The Effectiveness of Data Visualization
The effectiveness of this process depends on the comprehension and retention of the information. Businesses should undertake a systematic approach to conduct qualitative and quantitative evaluations. To achieve this, they can take the following steps:
Conduct usability testing: It is important to calculate an ideal sample size from the audience and have them interact with the visualization. This exercise should be done to observe how quickly they can draw insights and whether they can navigate the visualization intuitively.
Quantitative feedback: Metrics such as engagement rates, time spent per view, level of interaction etc., can be used to gather qualitative feedback about the visualization. These metrics can help businesses measure user attention capabilities.
Qualitative feedback: Businesses would do well to conduct surveys and interview the audiences to understand the perception created by the visualization. The questions in these surveys should pertain to its clarity, relevance and usefulness.
Platform testing: The visualizations also need to be tested on different platforms to ensure consistency. This testing should get increasingly rigorous if there is a higher chance of audiences using different platforms or devices.
Monitor long-term effectiveness: For long-term goals, visualizations should go through periodic reviews to measure periodic effectiveness. This process could involve tracking changes in user interactions and reevaluation of relevance as per evolving business needs.
Iterative improvements: This should happen in two scenarios. Different rounds of feedback should be used to make improvements in the visualization design and data. Secondly, changes must be made based on the results of long-term effectiveness testing, when indicated necessary.
By systematically evaluating these aspects, a business can not only measure the effectiveness of its current data visualizations but also gain insights that drive improvements in future visual analytics endeavors.
Real-World Use Cases for Data Visualization
The importance of data visualization is evident across various fields to harness its capabilities for enhanced understanding, decision-making and communication of complex information:
Healthcare
Healthcare extensively utilizes data visualization in patient care, population management and medical research. For instance, Florence Nightingale’s coxcomb chart demonstrates how data visualization shaped the correlation between sanitary conditions and mortality rates. In modern healthcare, it monitors vital signs, allowing for faster detection of changes in physiological data, signaling intervention needs.
Healthcare providers use various visualization techniques to identify trends, outliers and patterns that raw data or summarized statistics might not reveal. Medical professionals employ data visualization to assess patient records, insurance claims and more. Hospitals utilize dashboards to monitor bed occupancy levels, staff numbers and emergency room waiting times. The tools also help improve hospital operations, resource allocation and patient flow optimization.
Business intelligence
Business intelligence (BI) employs data visualization to summarize large data sets into digestible and visible insights. Businesses track and analyze sales data through data visualization to identify trends, successes and improvement areas. These analyses encompass overall sales dashboards highlighting monthly sales, regional performance and LoB (line of business) success. It also aids in understanding customer demographics and purchasing behaviors to enhance the effectiveness of marketing campaigns.
Visual representations promote cross-departmental communication and provide self-service analytics. Real-time access simplifies KPI tracking to measure organizational performance. These visualizations highlight the importance of data governance and security in leveraging data effectively for informed decision-making at every level of an organization.
Finance
Visuals simplify complex financial data, informing stakeholders about the financial health and operations of the organization. Management reports fill data visualization in quarterly or annual statements with breakdowns of revenues, expense analysis and profit trends. The development of risk management strategies benefits from risk assessment, stress test outcomes and credit exposure visualizations.
Data-driven illustrations aid in understanding and tracking financial risks, assisting fintech companies to provide data-driven investment recommendations to their customers. Financial data visualizations are also evolving towards more interactive, predictive and immersive techniques. Real-time streaming, mobile-friendly designs and ethical considerations in handling financial data are becoming increasingly prominent.
Retail and e-Commerce
Visual representations of sales data across different product categories, geographic regions or customer segments help businesses identify patterns, seasonal trends and areas for growth. Simultaneously, decisions based on inventory and demand forecast can prevent stockouts, reduce overstocking and streamline supply chain operations.
By visualizing interactions across various touchpoints, retailers can formulate targeted marketing campaigns, provide personalized recommendations and offer loyalty programs. The use of data visualization extends to website performance analysis. Graphical representation of click-through rates, bounce rates and conversion funnels allow businesses to optimize website design and improve user experience.
Competitive analysis through data visualization informs pricing strategies. Visual insights derived from pricing data, sales and customer behavior enable dynamic pricing adjustments and effective promotional campaigns to maximize profitability. Overall, data visualization empowers retailers to make informed decisions, improve operations and stay competitive.
Visualization Capabilities in Intellicus
Intellicus excels in business intelligence by offering a robust suite of visualization capabilities. The platform provides interactive and customizable dashboards for crafting impactful visual narratives. A diverse array of chart types, from bar graphs to heatmaps, allow Intellicus to deliver versatile data representation. It is also highly customizable for users to tailor visualizations to match branding and data emphasis.
The platform facilitates in-depth analysis through drill-down and drill-through functionalities for exploring data intricacies. It ensures cross-platform compatibility with seamless visualization sharing across web browsers, mobile devices and other applications. Moreover, Intellicus can source information from disparate data sources for unified access. Interactive elements within visualizations enhance user engagement and provide comprehensive data exploration. The platform also supports easy file export via scheduled reports, email or other applications.
With the latest 6.0 update, Intellicus now offers advanced capabilities by integrating an OLAP Engine and Executive Dashboards for deeper insights. Intellicus supports real-Time data visualizations of over 5000 events per second from various data streaming sources. It integrates with data science platforms to incorporate advanced predictive analytics in business reporting. These new-age features underscore Intellicus’ versatile and advanced data visualization capabilities for diverse business needs.
Data Visualization Best Practices
A data visualization effort can be considered successful only if the presented information conveys the right message and reveals useful insights. Along with the broader considerations in the process, designers should pay close attention to every minor detail. There is no official guidebook, but here are some data visualization best practices:
Define the objective:
Every visualization serves a purpose and it should be defined in detail. The designer should have a clear answer for the “why” of the process.
Keep it simple:
The data is already complex enough, which is the reason for the entire exercise. Therefore, it is imperative for visualization to communicate insights accurately and effectively. Data overload can defeat this purpose.
Use visual cues:
A visual presentation should have the correct aesthetics and signals to point the viewers in the right direction. Using different colors and shapes to represent distinct data points is an accepted practice.
Choose the right chart:
The choice of chart depends on the most important KPI. From a range of visualizations, designers need to pick the right design to highlight variables like frequency, density, distribution, comparison or volume.
Review and revise:
Seek feedback from relevant sources and create iterations of your visualization. Incremental updates can lead to improvement and effectiveness.
Open-Source Data Visualization Tools
Open-source data visualization tools play a pivotal role in transforming complex data into understandable, interactive and visually engaging formats. These tools are essential in various domains such as business intelligence, scientific research and data journalism, catering to a broad spectrum of users. These are the most prominent tools and their applications:
D3.js: Developers use this JavaScript library’s flexibility to create highly sophisticated visualizations such as interactive graphs and Geo maps. It excels in developing browser-based dynamic and interactive data visualizations compatible with HTML, SVG and CSS. D3.js is most used for web-based visualization projects, academic research and business analytics dashboards.
Python Libraries (Matplotlib and Seaborn): Matplotlib, a low-level plotting library written in Python, incorporates visualizations into various applications using PNG, PDF, SVG, EPS and other similar formats. This library supports scientific computing by creating static, animated and interactive visualizations through Python scripts, web application servers and GUI toolkits.
Seaborn, a derivative of Matplotlib, is used extensively in academic research for creating statistical graphics and complex data visualizations.
R Libraries (ggplot2 and Shiny): ggplot2 in R offers a systematic approach for graph creation, known for its extensibility and customization. Researchers and users praise its ability to craft complex graphics like bar charts, line graphs and scatter plots.
On the other hand, Shiny enables users to build interactive web applications directly from R, eliminating the need for extensive HTML, CSS or JavaScript knowledge. It is commonly used for developing data analysis and visualization web applications, particularly in business intelligence and data science contexts.
Plotly: Compatible with Python, R and JavaScript, developers use Plotly for a wide range of chart types, including 3D graphs and interactive features like zooming and panning. It integrates well with various data science languages and is a staple in academia and industry for creating interactive visualizations, dashboards and data applications.
Bokeh: Widely utilized in financial, statistical and social sciences, this Python library uses HTML and JavaScript to render graphics, suitable for interactive plots and dashboards on web pages, creating a range of interactive charts and data applications on the internet.
Apache Superset: A scalable web application for data discovery and visualization, Apache Superset supports SQL querying of several SQL-based data sources. It finds its primary application in business intelligence, aiding companies in visualizing data and creating interactive dashboards.
Grafana: Known for querying and visualizing time series data, Grafana works with data sources like Prometheus and Elasticsearch. It is used in IoT device network administration, network monitoring and server infrastructure management.
The unique features and strengths of each of these tools make them well-suited for different data visualizations. Organizations need to select the best-suited tool considering project needs, technical knowledge and the type of data.
The Future of Data Visualization
Data visualization is evolving towards augmented analytics, immersive experiences and collaborative contributions. This technological improvement not only makes data easier to understand but also changes our perception and interaction with it. The future promises several value additions, such as:
Enhancing collaborative data exploration
Project teams in the future will use this discipline for real-time data analysis, providing a broader knowledge base and immersive experience. The integration of popular communication platforms with data visualization tools creates forums for easier sharing and discussion, fostering a more inclusive data-driven culture in organizations.
Embracing augmented analytics
The convergence of ML and natural language processing (NLP) will automatically prepare data for intuitive exploration, facilitating automated insight generation and broadening data analysis to a wider audience. Future data visualization tools will most likely have advanced AI algorithms to automatically identify major trends and anomalies in data, saving time and expertise for deeper analysis.
Focus on contextual visualization
Future developments may include context-aware systems adapting visualizations according to user context, whether it is their location, time or specific task. Personalized data narratives involve tailoring data stories to diverse needs based on individual preferences, making data more relatable.
Immersive experiences in mixed reality
These visualizations blend virtual and augmented reality, rendering complex data sets more tangible and accessible, altering how we perceive data. Intuitive comprehension of complex concepts in fields like education, engineering and healthcare will be facilitated through immersive data visualizations.
Advanced analytical capabilities
The next generation of data visualization tools will not only predict tendencies but also offer advice on actions, resulting in better decisions when combined with visualization. Tools will advance to live data stream management, especially in fast-paced industries requiring real-time insights.
Ethical and responsible visualization
The increasing use of data visualization in decision-making necessitates ethical considerations in representation, privacy and consent for data usage. Addressing biases in data presentation will be a key focus, involving the design of algorithms and practices that detect and correct biases.
Conclusion
Data visualization, a fusion of data science and creative design, involves multiple stages from data collection to crafting informative visual narratives. As data generation accelerates, selecting appropriate visual elements and design principles becomes crucial. Storytelling through visualization is pivotal across various fields, aiding in communicating complex information in an engaging and understandable manner.
Incorporating AI and ML in data visualization automates data analysis, enhancing predictive capabilities and providing interactive experiences. However, ensuring transparency and ethical considerations are vital in this domain. Data visualization skills are crucial to translate intricate information into comprehensible visual stories. The future aims for an interactive, AI-driven and user-centric experience.